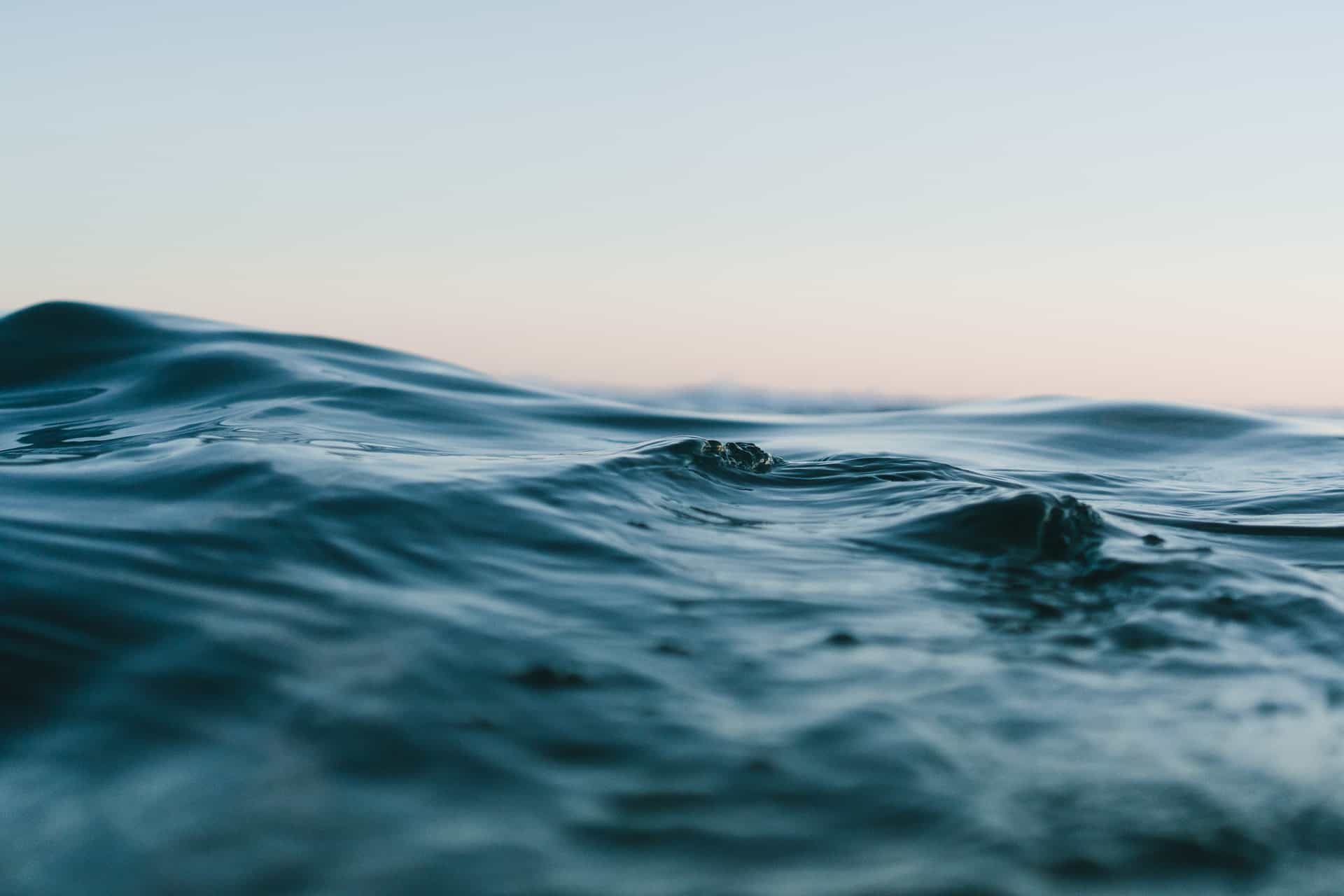
Part I - Toward NNGP and NTK [draft]
Neural Network Gaussian Process(NNGP) Model the neural network as GP, aka neural network Gaussian Process(NNGP). Intuitively, the kernel of NNGP compute the distance between the output vectors of 2 input data points. We define the following functions as neural networks with fully-conntected layers: $$z_{i}^{1}(x) = b_i^{1} + \sum_{j=1}^{N_1} \ W_{ij}^{1}x_j^1(x), \ \ x_{j}^{1}(x) = \phi(b_i^{0} + \sum_{k=1}^{d_{in}} \ W_{ik}^{0}x_k(x))$$ where $b_i^{1}$ is the $i$th-bias of the second layer(the same as first hidden layer), $W_{ij}^{1}$ is the $i$th-weights of the first layer(the same as input layer) , function $\phi$ is the activation function, and $x$ is the input data of the neural network....